February 19 - 21
Seven Springs, PA
Visit our booth
Meet Lunaphore
Connect with our experts in person and explore our comprehensive spatial biology solutions, designed to support the scientific community throughout all stages — from early discovery to translational research. Experience the revolutionary same-section multiomics on COMET™: fully-automated, protease-free RNA and protein detection.
Are you interested in learning more about our spatial biology solutions? Reach out to us to arrange a meeting with our team.
Stop by the Lunaphore booth to browse raw multiomics images on various tissue types. Book a meeting with our team to learn how top-notch laboratories, biopharma and CROs leverage COMET™.
Dec 11 – 12
Booth #56
BOOK A MEETING WITH LUNAPHORE →
(Clicking on the link you will be able to select your preferred meeting time)
Poster Presentations
December 11 - 12
Exhibition Hall - Poster 21
Identifying biomarkers that predict patient response to immunotherapy is critical for optimizing treatment strategies and improving clinical outcomes. Here, we present a novel multiplex imaging-based immunotherapy panel and a comprehensive analysis pipeline to characterize the spatial distribution and function of immune cells and its application for spatial biomarkers detection in a cohort of immunotherapy and targeted therapy-treated melanoma patients.
We designed a 28-plex panel to perform sequential immunofluorescence (seqIF™) on the COMET™ platform (PMID: 37813886) to target key biomarkers associated with tumor microenvironment composition (TME), immune cell infiltration and immune checkpoint pathways. Utilizing Nucleai’s deep-learning-based multiplex imaging analysis pipeline (Markovits, bioRxiv), we identified 13 cell types, including 9 different immune cell populations, in addition to 10 cell state markers. Cells were assigned to the tumor area or TME and spatial features were calculated based on cell type, marker positivity, and area assignments. We obtained pre-treatment biopsies from patients with a known long-term response or rapid progression to immunotherapy combination treatment (Ipilimumab+Nivolumab) from the SECOMBIT Phase II Trial (NCT02631447, PMID: 36049147, PMID: 38167503). We profiled these samples using the aforementioned panel and analysis solution. We aimed to identify spatial biomarkers that can differentiate between long-term responders and non-responders to immunotherapy.
Our novel multiplex imaging panel and analysis pipeline demonstrated high balanced accuracy (> 0.8) and F1 scores (> 0.8) in cell typing and protein quantification for the majority of cell types and markers. This analysis pipeline enables the quantification of known biomarkers such as T cell activation states, T cell infiltration patterns, and TLS maturation. In addition, we explored several additional biomarkers, such as receptor-ligand interactions of PD-1 and PD-L1, interactions between T cells and other immune populations, and stromal cells or tumor cells as additional biomarkers for their association with patient outcomes.
The development of this multiplex imaging panel and deep learning pipeline highlights the potential of integrating multiplex imaging with AI analysis to enhance our understanding of treatment efficacy and resistance mechanisms, ultimately aiming to improve patient outcomes in clinical practice.
Speaker
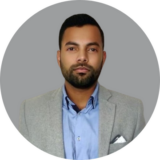
Wollis Vas, Ph.D.
Scientific Market & Translational Strategy Manager
Lunaphore
December 11 - 12
Exhibition Hall - Poster 23
Spatial hyperplex immunofluorescence enables the visualization of cellular and non-cellular tissue components simultaneously. However, the increasing number of biomarkers detected by spatial proteomics quickly escalates the complexity of images and renders their interpretation challenging. Extracting quantitative data from images remains a challenge as it requires extensive training and experience in data analysis. Additionally, inferring cellular interactions and dependencies from spatial assays is an important milestone that needs to be overcome with image analysis approaches.
Here, we present an end-to-end solution that combines automated hyperplex execution with image data extraction to study the spatial composition of TME.
Sequential multiplex immunofluorescence (seqIF™) was performed on COMET™ on an FFPE Breast Tissue Microarray (TMA), generating images containing 42 layers: nuclear DAPI, tissue autofluorescence, and 39 single layers for biomarkers. All layers were delivered as a single ome.tiff file and processed using HORIZON™ image analysis software in combination with downstream statistical analysis. The tissue composition was interrogated with the use of an in-house trained nuclei detection algorithm (Schmid et al., MICCAI 2018; Weigert et al., WACV 2020), in-house developed image artifact exclusion approach, and spatial analysis based on a Squidpy workflow (Palla G. et al., Nat Meth 2022).
We interrogated the composition of breast tumor tissues (n=9). Our in-house developed pipeline allows for filtering out both autofluorescent objects and segmentation artifacts based on the tissue autofluorescence and morphological features. Once the quality of segmented cell detection was assured, over 20 different cell phenotypes were identified by unsupervised Leiden clustering and visualized using UMAP dimensionality reduction method. It revealed several myeloid cell subsets highlighting their heterogeneous phenotypes including, but not limited to, immunosuppressive neutrophils and macrophages. Contrastingly, the uniform presence of T regulatory lymphocytes was detected in majority of cores and their co-occurrence with CD14+CD163+ macrophages was observed.
This workflow is easily transferrable to various cohorts of specimens to provide a toolset for spatial cellular dissection of the cancer milieu.
Speaker
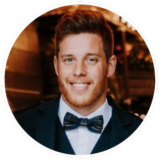
William McTavish
Technical Sales Specialist
Lunaphore